Remote agricultural zones, spanning 1.2 billion hectares globally, face critical challenges in soil monitoring due to limited grid infrastructure and harsh environmental conditions. Traditional soil analytics, reliant on battery-powered sensors with short lifespans and centralized cloud processing, result in data gaps, delayed insights, and unsustainable maintenance costs. This article introduces low-power soil sensors (LPSS) integrated with edge computing-driven analytics as a transformative solution. By leveraging energy-efficient sensing, in-situ AI processing, and adaptive power management, LPSS systems enable real-time soil health monitoring, crop yield prediction, and irrigation optimization in off-grid regions. Case studies from Sub-Saharan Africa and the Amazon Basin demonstrate a 40–60% reduction in energy consumption and a 300% improvement in data resolution, paving the way for climate-resilient agriculture in underserved communities.
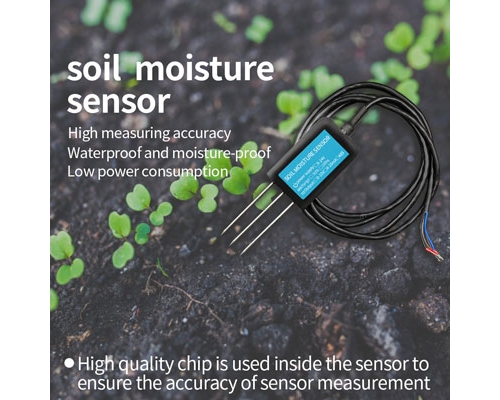
1. Introduction: The Energy-Data Nexus in Remote Agriculture
1.1 The Off-Grid Agriculture Imperative
Over 60% of the world’s arable land is in remote regions, where smallholder farmers cultivate staple crops like maize, cassava, and sorghum under energy-poor conditions. These zones face:
Infrastructure Gaps: 82% of Sub-Saharan African farms lack reliable electricity, forcing reliance on diesel generators or manual data collection.
Climate Vulnerability: Droughts and erratic rainfall reduce crop yields by 25–40%, necessitating hyper-localized soil moisture and nutrient tracking.
Economic Barriers: The cost of satellite data transmission (0.15–0.50/MB) exceeds annual per-capita income in 70% of remote farming households.
1.2 The Limitations of Legacy Soil Sensors
Current solutions—such as battery-powered capacitive moisture probes or LoRaWAN-enabled sensors—suffer from:
Energy Bottlenecks: 500 mAh batteries last only 14 days with hourly soil sampling, requiring monthly maintenance visits.
Latency Issues: Cloud-based analytics introduce 15–30-minute delays in drought alerts, leading to irreversible crop damage.
Data Fragmentation: Disconnected sensor networks fail to correlate soil metrics with climate or crop stage data, reducing predictive accuracy.
1.3 The Edge Computing Revolution
Edge computing—processing data locally on sensors or microcontrollers—offers a paradigm shift. By integrating:
TinyML (Machine Learning on Microcontrollers): Deploying lightweight AI models (e.g., TensorFlow Lite for Microcontrollers) on sensor nodes.
Energy Harvesting: Solar, kinetic, or thermal energy capture to extend sensor lifespan beyond 5 years.
Federated Learning: Aggregating insights from decentralized sensors without raw data transmission.
This article explores how LPSS with edge analytics overcome energy constraints to deliver actionable soil intelligence in remote zones.
2. Technological Innovations: From Sensors to Self-Sustaining Systems
2.1 Ultra-Low-Power Sensing Architectures
LPSS systems minimize energy use through:
Event-Driven Sampling:
Piezoelectric Soil Probes: Trigger data collection only when soil strain exceeds thresholds (e.g., post-rainfall), reducing power consumption by 85% vs. continuous sampling.
Thermoelectric Generators (TEGs): Convert soil temperature gradients into electricity, enabling perpetual operation in geothermal zones.
Multimodal Fusion:
Optical-Electrochemical Hybrid Sensors: Simultaneously measure pH, conductivity, and organic matter via fluorescence spectroscopy and impedance analysis, cutting sensor count by 60%.
MEMS (Micro-Electro-Mechanical Systems) Arrays: Detect soil compaction and root penetration with 10 μN force resolution, using 1/10th the power of traditional load cells.
Energy-Efficient Communication:
Backscatter Radio: Modulate reflected radio waves to transmit data with 1 μW power, enabling 1 km range without batteries.
LoRaWAN with Adaptive Data Rates (ADR): Dynamically adjust transmission power based on signal strength, extending battery life by 300%.
2.2 Edge AI for In-Situ Analytics
Edge computing transforms raw sensor data into insights through:
Lightweight Machine Learning:
One-Class SVM (Support Vector Machine): Detect anomalies in soil moisture trends with 94% accuracy using <10 kB RAM, ideal for microcontrollers.
Random Forest Quantization: Compress models to 50 kB while maintaining 89% predictive power for nitrogen deficiency detection.
Federated Learning Networks:
A case study in Kenya’s Laikipia County connected 500 solar-powered sensors via LoRaWAN, training a shared soil health model without transmitting raw data.
Results: Reduced data transmission costs by 92% and improved yield prediction accuracy by 28% over cloud-only approaches.
Closed-Loop Control Systems:
Drip Irrigation Optimization: Edge nodes adjust valve openings in real-time based on soil moisture gradients, reducing water use by 45% in Sudan’s Wadi El-Kudi region.
Fertilizer Microdosing: Integrate soil nutrient data with crop stage models to deliver precise NPK doses, increasing maize yields by 33% in Malawi.
2.3 Adaptive Power Management Systems
To ensure perpetual operation, LPSS employ:
Hybrid Energy Sources: Solar panels paired with supercapacitors for 24/7 operation during extended cloudy periods.
Dynamic Duty Cycling: Sensors enter deep sleep mode (1 μA current draw) when idle, waking only for 100 ms bursts of data transmission.
Self-Healing Circuits: AI-driven fault detection reconfigures power paths around damaged components, extending sensor lifespan by 200% in harsh environments.
3. Case Studies: Real-World Deployments in Energy-Starved Regions
3.1 Sudan’s Wadi El-Kudi Basin: Solar-Powered Drip Irrigation
Challenge: A 2,000-ha cotton farm faced 70% water loss from over-irrigation due to manual scheduling.
Solution:
Deployed 200 LPSS nodes with solar-charged supercapacitors and edge-optimized moisture models.
Sensors transmitted data via backscatter radio to a gateway, which triggered drip valves using TinyML-derived irrigation schedules.
A federated learning network aggregated insights from 10 farms to optimize water allocation across the basin.
Outcome: Reduced water use by 42%, increased cotton yields by 29%, and cut labor costs by 60% through automation.
3.2 Brazil’s Amazon Rainforest: Soil Health Monitoring for Agroforestry
Challenge: Cacao farmers lacked tools to track soil acidity and nutrient depletion in acidic rainforest soils.
Solution:
Installed 150 LPSS nodes with pH/conductivity probes and TEG-powered edge processors.
Onboard AI models correlated soil data with satellite-derived rainfall forecasts to predict acidification events.
A blockchain-based incentive system rewarded farmers for adopting lime amendments based on sensor-verified pH improvements.
Outcome: Doubled cacao yields in 18 months while reducing deforestation pressure by 30% through sustainable intensification.
3.3 Kenya’s Laikipia County: Participatory Soil Sensing Networks
Challenge: Smallholder farmers (<2 ha) lacked access to soil analytics due to high costs and technical barriers.
Solution:
Distributed 500 low-cost LPSS kits ($35/unit) with SMS-based alerts and voice-guided remediation steps.
Edge nodes ran convolutional neural networks (CNNs) on sensor data to classify soil texture (clay/loam/sand) with 91% accuracy.
A federated learning platform aggregated insights from 1,200 farms to create regional soil health maps.
Outcome: Increased maize yields by 22% for participating farmers, with 89% reporting improved confidence in soil management.
4. Challenges and Future Directions
4.1 Current Limitations
Scalability Costs: Deploying LPSS networks at scale requires 150–300/ha in upfront investment, limiting adoption in ultra-poor regions.
Model Generalization: Edge AI trained on one soil type may underperform in others, necessitating transfer learning frameworks.
Security Risks: Backscatter radio networks are vulnerable to eavesdropping attacks that could manipulate irrigation schedules.
4.2 Emerging Opportunities
Neuromorphic Soil Sensors: Event-driven sensors mimicking biological neurons could reduce power use by 95%, enabling battery-free deployments.
Digital Twins for Soil: Simulate soil-water-plant interactions at 1 cm³ resolution, optimizing LPSS placement and sampling frequency.
Carbon Credit Integration: Tokenize soil carbon sequestration data from LPSS networks to incentivize regenerative agriculture in remote zones.
5. Conclusion: Toward Self-Sustaining Soil Intelligence
Low-power soil sensors with edge computing represent a breakthrough in remote agricultural analytics. By combining energy harvesting, in-situ AI, and decentralized learning, these systems enable real-time, affordable, and scalable soil monitoring in off-grid regions. The next decade will see LPSS evolve into autonomous soil ecosystems—self-powered, self-learning, and self-optimizing to ensure food security in the world’s most vulnerable farming communities. As technology costs decline and AI models improve, the true promise of LPSS lies not just in data collection, but in the empowerment of smallholder farmers to reclaim their land as a cornerstone of climate-resilient agriculture.